
Research
My research focuses on the ways that technology mediates knowledge, understanding, and our practice of giving and receiving explanations. 

I explore the theoretical and philosophical foundations of scientific explanation and their potential for enabling understanding. I then apply these theoretical foundations toward use cases in computer science and human computer interaction, such as explanatory frameworks for decision support systems and norms of information sharing on social media. (Pre-prints available at philpapers)
Below are a selection of my papers.

Mark I perception 1960, Cornell Aeronautical Laboratory
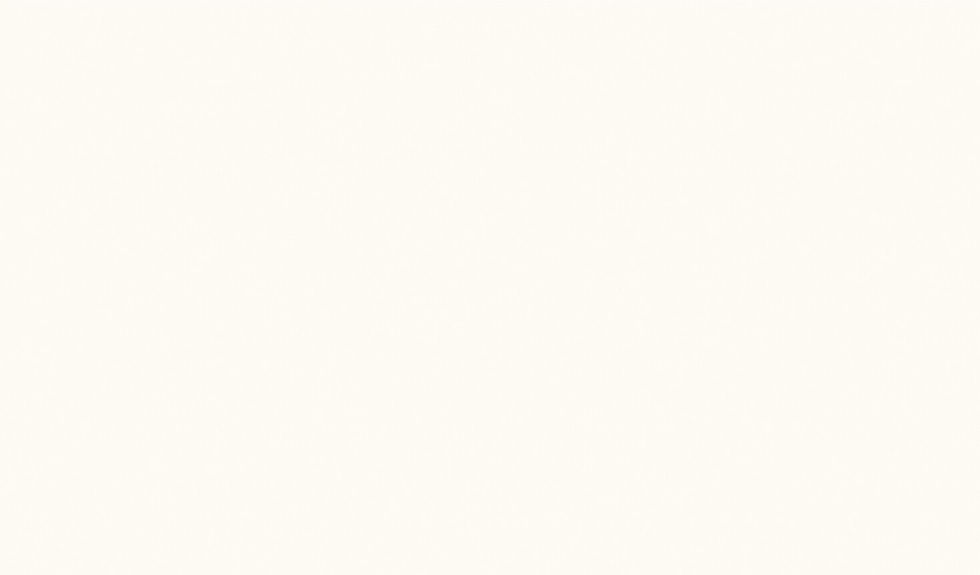
Machine Learning
British Journal for the Philosophy of Science (2022)
Honorable Mention for 2022 BJPS Popper Prize
Using the case of deep neural networks, I argue that it is not the complexity or black-box nature of a model that limits how much understanding the model provides. Instead, it is the presence of link uncertainty, a lack of scientific and empirical evidence supporting the link that connects a model to the target phenomenon, that primarily prohibits understanding.
Thomas Grote, Konstantin Genin, and Emily Sullivan
Philosophy Compass (2024)
Issues of reliability are claiming center-stage in the epistemology of machine learning. This paper unifies different branches in the literature and points to promising research directions, whilst also providing an accessible introduction to key concepts in statistics and machine learning – as far as they are concerned with reliability.
Proceedings of the 2024 ACM Conference on Fairness, Accountability, and Transparency (FAccT’ 24)
Drawing on the use of idealizations in the natural sciences and philosophy of science, I introduce a novel framework for evaluating whether xAI methods engage in successful idealizations or deceptive explanations (SIDEs). SIDEs evaluates whether the limitations of xAI methods, and the distortions that they introduce, can be part of a successful idealization or are indeed deceptive distortions as critics suggest.
Philosophy of Science (2023)
I argue that machine learning (ML) models used in science function as highly idealized toy models. If we treat ML models as a type of highly idealized toy model, then we can deploy standard representational and epistemic strategies from the toy model literature to explain why ML models can still provide epistemic success despite their lack of similarity to their targets.
Philosophy of Science (2022)
Under what conditions does machine learning (ML) model opacity inhibit the possibility of explaining and understanding phenomena? In this article, I argue that non-epistemic values give shape to the ML opacity problem even if we keep researcher interests fixed. Treating ML models as an instance of doing model-based science to explain and understand phenomena reveals that there is (i) an external opacity problem, where the presence of inductive risk imposes higher standards on externally validating models, and (ii) an internal opacity problem, where greater inductive risk demands a higher level of transparency regarding the inferences the model makes.
Emily Sullivan and Philippe Verreault-Julien
AIES '22: Proceedings of the 2022 AAAI/ACM Conference on AI, Ethics, and Society
People are increasingly subject to algorithmic decisions, and it is generally agreed that end-users should be provided an explanation or rationale for these decisions. There are different purposes that explanations can have, such as increasing user trust in the system or allowing users to contest the decision. One specific purpose that is gaining more traction is algorithmic recourse. We first propose that recourse should be viewed as a recommendation problem, not an explanation problem. Then, we argue that the capability approach provides plausible and fruitful ethical standards for recourse. We illustrate by considering the case of diversity constraints on algorithmic recourse. Finally, we discuss the significance and implications of adopting the capability approach for algorithmic recourse research.